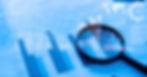
In the fast-paced world of manufacturing, the ability to identify and address root causes of issues is crucial for maintaining efficiency, quality, and overall operational excellence. Traditional methods of root cause analysis often involve manual inspection and complex data interpretation, leading to time-consuming processes and potential human errors. However, with the advent of advanced technologies, automated data analysis has emerged as a game-changer in the manufacturing sector. In this article, we'll explore the various types of data analysis that can be automated for root cause analysis, revolutionizing the way manufacturers address challenges and optimize their processes.Â
Â
1. Statistical Process Control (SPC) AutomationÂ
Â
Statistical Process Control is a cornerstone of quality management in manufacturing. Automation of SPC involves the continuous monitoring of key process parameters using statistical methods. Automated data analysis tools can quickly identify variations, trends, and anomalies in production data, allowing manufacturers to pinpoint potential root causes of deviations from optimal performance. SPC automation not only improves the speed of analysis but also enhances the accuracy of identifying issues affecting product quality.Â
Â
2. Machine Learning Predictive AnalyticsÂ
Â
Machine learning (ML) algorithms have become increasingly sophisticated in predicting outcomes based on historical data. In manufacturing, predictive analytics can be employed to automatically analyze vast datasets and identify patterns that may lead to issues. By training ML models to recognize correlations between variables, manufacturers can predict potential root causes before they escalate into significant problems. This proactive approach minimizes downtime, reduces waste, and optimizes production processes.Â
Â
3. Failure Mode and Effects Analysis (FMEA) AutomationÂ
Â
Failure Mode and Effects Analysis is a systematic method for evaluating processes to identify where and how they might fail and assess the relative impact of different failures. Automation of FMEA involves the use of software tools to analyze historical failure data, process parameters, and performance metrics. By automating this analysis, manufacturers can swiftly identify high-risk areas in their processes, enabling them to prioritize improvements and allocate resources effectively to address potential root causes.Â
Â
4. Root Cause Analysis (RCA) AlgorithmsÂ
Â
Traditional Root Cause Analysis involves a manual, time-consuming process of investigating potential causes of issues. However, automation using advanced algorithms can significantly expedite this process. These algorithms can analyze historical and real-time data, applying logical reasoning to identify the most probable root causes of problems. By automating the RCA process, manufacturers can reduce the time spent on troubleshooting, leading to quicker resolutions and improved overall operational efficiency.Â
Â
5. Real-time Monitoring and ControlÂ
Â
Automated real-time monitoring and control systems are instrumental in identifying and addressing root causes as they occur. These systems continuously collect data from various sensors and equipment on the factory floor, instantly analyzing the information for anomalies. If any deviation from the norm is detected, the system can trigger alerts and even initiate corrective actions autonomously. This real-time responsiveness minimizes the impact of issues and enhances the overall reliability of manufacturing processes.Â
Â
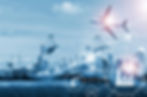
6. Supply Chain Data IntegrationÂ
Â
The manufacturing process is intricately connected to the supply chain, and disruptions in the supply chain can have a cascading effect on production. Automated data analysis can integrate information from the entire supply chain, including suppliers and logistics. By continuously monitoring this integrated data, manufacturers can identify potential disruptions or delays in the supply chain that may impact production. Addressing these issues early on can prevent downtime and production bottlenecks.Â
Â
7. Energy Consumption AnalysisÂ
Â
Manufacturing facilities often consume significant amounts of energy, and analyzing energy usage data can reveal insights into operational efficiency and potential root causes of inefficiencies. Automated data analysis tools can track energy consumption patterns, identify areas of excessive usage, and suggest optimizations. By addressing energy-related root causes, manufacturers not only improve sustainability but also reduce operational costs.Â
Â
8. Human-Machine Interface (HMI) AutomationÂ
Â
Automation is not limited to machines; it extends to the interfaces through which humans interact with machines and systems. HMI automation involves the analysis of data collected from human-machine interactions. This includes monitoring operator inputs, system responses, and feedback. By automating the analysis of HMI data, manufacturers can identify human-related errors, training needs, and ergonomic issues, contributing to a safer and more efficient work environment.Â
Â
ConclusionÂ
Â
In conclusion, the integration of automated data analysis into root cause analysis processes is revolutionizing manufacturing. From statistical process control to machine learning predictive analytics, the tools available today enable manufacturers to identify and address root causes with unprecedented speed and accuracy. Embracing automation in data analysis not only enhances operational efficiency but also positions manufacturers to stay competitive in an increasingly dynamic and complex global market. As the manufacturing industry continues to evolve, the adoption of automated data analysis for root cause analysis will play a pivotal role in shaping the future of efficient and sustainable production processes.Â