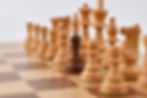
Manufacturing success depends on maintaining optimal production efficiency. Anomaly detection, powered by advanced technologies, has emerged as a game-changer in identifying irregularities and potential issues in the manufacturing process. Here, we explore four key points that elucidate how anomaly detection works in the manufacturing realm.
Sensor Data Monitoring for Real-Time Insights:Â
Anomaly detection relies heavily on the continuous monitoring of sensor data throughout the manufacturing process. Sensors strategically placed across production lines capture a plethora of data related to temperature, pressure, vibration, and other critical parameters. These data points create a real-time snapshot of the manufacturing environment. Anomaly detection algorithms then analyze this data, seeking patterns and deviations that may indicate faults or irregularities in the machinery or production process.Â
Â
Pattern Recognition and Machine Learning Algorithms:
At the core of anomaly detection in manufacturing is the use of advanced machine learning algorithms capable of recognizing patterns and abnormalities within vast datasets. These algorithms are trained on historical data, learning the normal patterns of operation. When deployed in real-time, they continuously compare incoming data against the established patterns. Deviations from the norm trigger alerts or notifications, signaling potential anomalies that require further investigation. This proactive approach allows manufacturers to identify issues before they escalate, minimizing downtime and optimizing production efficiency.Â
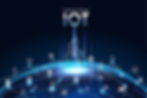
Â
Predictive Maintenance for Minimizing Downtime:
Anomaly detection in manufacturing goes beyond merely identifying irregularities; it extends to predictive maintenance. By analyzing patterns of equipment behavior, anomaly detection systems can forecast potential machinery failures or maintenance needs. This predictive capability empowers manufacturers to schedule maintenance activities precisely when needed, minimizing unplanned downtime and reducing the risk of costly breakdowns. Predictive maintenance enhances overall equipment effectiveness and extends the lifespan of machinery, contributing to sustained operational efficiency.Â
Â
Integration with IoT and Edge Computing:
The integration of anomaly detection with the Internet of Things (IoT) and edge computing amplifies its effectiveness in manufacturing. IoT devices gather data from various endpoints, and edge computing processes this data in real-time, reducing latency. Anomaly detection algorithms can be deployed at the edge, allowing for quicker analysis and response. This integration ensures that potential anomalies are identified swiftly, providing a rapid and targeted response to deviations from normal operating conditions.Â
Â
In summary, anomaly detection in manufacturing is a sophisticated process that leverages sensor data, machine learning algorithms, predictive maintenance strategies, and seamless integration with IoT and edge computing. By continuously monitoring and analyzing vast datasets, anomaly detection systems empower manufacturers to identify irregularities, predict potential issues, and proactively address maintenance needs. This precision in production not only minimizes downtime and operational disruptions but also contributes to a more efficient, cost-effective, and sustainable manufacturing environment.Â